일 | 월 | 화 | 수 | 목 | 금 | 토 |
---|---|---|---|---|---|---|
1 | 2 | 3 | 4 | 5 | 6 | 7 |
8 | 9 | 10 | 11 | 12 | 13 | 14 |
15 | 16 | 17 | 18 | 19 | 20 | 21 |
22 | 23 | 24 | 25 | 26 | 27 | 28 |
29 | 30 |
- Python
- object detection
- reinforcement learning
- computer vision
- coding test
- 백준
- 알고리즘
- 프로그래머스
- 논문
- 내용추가
- 모두를 위한 딥러닝
- Today
- Total
목록전체 (41)
NISSO
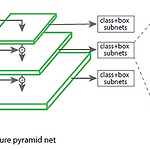
Focal Loss for Dense Object Detection (2017) https://arxiv.org/abs/1708.02002 Focal Loss for Dense Object Detection The highest accuracy object detectors to date are based on a two-stage approach popularized by R-CNN, where a classifier is applied to a sparse set of candidate object locations. In contrast, one-stage detectors that are applied over a regular, dense sampl arxiv.org RetinaNet은 Clas..
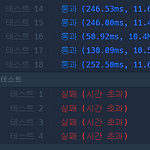
def solution(info, query): info = [i.split() for i in info] query = [q.split(' and ') for q in query] answer = [] for lang, pos, car, x in query: soul, scr = x.split() cnt = 0 for i in info: qr = [lang, pos, car, soul] if '-' in qr: for j in range(4): if qr[j] == '-': qr[j] = i[j] if qr == i[:-1] and int(i[-1]) >= int(scr): cnt += 1 answer.append(cnt) return answer 위와 같이 푼 결과는 시간 초과.. 문제에 대한 질문들..
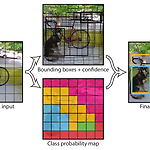
YOLO:: You Only Look Once:Unified, Real-Time Object Detection (2016) https://arxiv.org/abs/1506.02640 You Only Look Once: Unified, Real-Time Object Detection We present YOLO, a new approach to object detection. Prior work on object detection repurposes classifiers to perform detection. Instead, we frame object detection as a regression problem to spatially separated bounding boxes and associated..
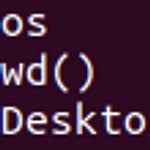
os 모듈은 OS(Operating System)를 제어할 수 있는 유용한 모듈이다. 프로젝트를 진행하면서 많이 사용한 함수를 정리해보려고 한다. import os 먼저 os 모듈은 임포트해준다. 1. os.getcwd() get curren working directory의 약자로, 현재 작업 디렉토리를 반환한다. 상대 경로 (ex. ../data/train/)를 지정하거나 현재 디렉토리를 확인할 때 유용하다. 리눅스 터미널 창에서 pwd 명령어와 같은 역할을 한다. 2. os.listdir('./') 인자로 './' 를 넣어주면 현재 디렉토리에 있는 모든 파일과 폴더를 보여준다. 이 때 리눅스 터미널 창에서 ls 명령어와 같은 역할을 한다. 결과는 리스트 형태로 주어지기 때문에, 하위 디렉토리의 더 ..
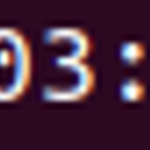
코드를 짜고 프로그램을 실행할 때, 전체나 일부 코드의 실행 시간을 알아야 할 때가 있다. 이럴 때, time과 datetime 라이브러리를 사용하여 쉽게 시간을 측정하고 출력할 수 있다. 원리는 간단하다. time.time()은 현재 시간을 계산해주는데, 각 코드를 실행하기 전후의 시간을 변수에 저장해놓고 빼주면 된다. # 코드1 import time import datetime start = time.time() y = function1(X) # 실행할 코드 end = time.time() sec = (end - start) result_list = str(datetime.timedelta(seconds=sec)).split(".") print(result_list[0]) 함수 function1이 있..
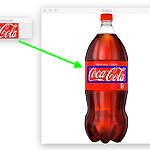
Template Matching (템플릿 매칭) object detection의 가장 단순하고 기본적인 형태로, 이미지 내에서 탐지하려는 객체가 포함된 '템플릿' 이미지를 찾는 것이다. 템플릿 이미지를 입력 이미지에서 슬라이딩시켜 전체 이미지에 대해 매칭하면서 두 이미지의 유사도를 계산한다. 이 때 openCV의 cv2.matchTemplate() 함수를 사용한다. cv2.matchTemplate(image, templ, method, result=None, mask=None) image : 입력 이미지 templ : 템플릿 이미지 method : 매칭 방법. 이에 따라 유사도 계산 방법이 달라진다. result : 매칭 결과 행렬. (W - w + 1) * (H - h + 1) 크기의 2차원 array..
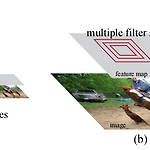
Faster R-CNN: Towards Real-Time Object Detection with Region Proposal Networks https://arxiv.org/abs/1506.01497 Faster R-CNN: Towards Real-Time Object Detection with Region Proposal Networks State-of-the-art object detection networks depend on region proposal algorithms to hypothesize object locations. Advances like SPPnet and Fast R-CNN have reduced the running time of these detection networks,..
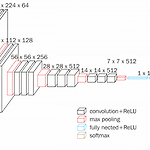
Very Deep Convolutional Networks for Large-Scale Image Recognition * 대규모 이미지 인식을 위한 아주 깊은 컨볼루션 신경망 * Computer Vision 분야에 대한 큰 가능성을 보여줌 * VGG : Visual Geometry Group, 옥스포드대 연구팀 이름 https://arxiv.org/pdf/1409.1556.pdf VGGNet은 CNN의 깊이에 따른 정확도를 연구할 목적으로 만든 이미지 인식 모델이다. Abstract 대규모 이미지 인식에서 convolutional network의 깊이가 정확도에 미치는 영향을 조사 3*3 convolution filter 구조를 사용해 깊은 신경망을 평가 16-19 weight layer의 깊이로 선행..