일 | 월 | 화 | 수 | 목 | 금 | 토 |
---|---|---|---|---|---|---|
1 | ||||||
2 | 3 | 4 | 5 | 6 | 7 | 8 |
9 | 10 | 11 | 12 | 13 | 14 | 15 |
16 | 17 | 18 | 19 | 20 | 21 | 22 |
23 | 24 | 25 | 26 | 27 | 28 | 29 |
30 | 31 |
- 알고리즘
- Python
- object detection
- 논문
- coding test
- 프로그래머스
- reinforcement learning
- 내용추가
- 백준
- 모두를 위한 딥러닝
- computer vision
- Today
- Total
목록computer vision (8)
NISSO
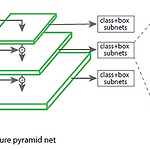
Focal Loss for Dense Object Detection (2017) https://arxiv.org/abs/1708.02002 Focal Loss for Dense Object Detection The highest accuracy object detectors to date are based on a two-stage approach popularized by R-CNN, where a classifier is applied to a sparse set of candidate object locations. In contrast, one-stage detectors that are applied over a regular, dense sampl arxiv.org RetinaNet은 Clas..
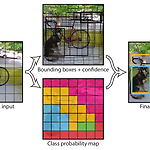
YOLO:: You Only Look Once:Unified, Real-Time Object Detection (2016) https://arxiv.org/abs/1506.02640 You Only Look Once: Unified, Real-Time Object Detection We present YOLO, a new approach to object detection. Prior work on object detection repurposes classifiers to perform detection. Instead, we frame object detection as a regression problem to spatially separated bounding boxes and associated..
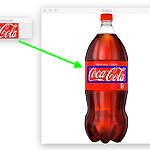
Template Matching (템플릿 매칭) object detection의 가장 단순하고 기본적인 형태로, 이미지 내에서 탐지하려는 객체가 포함된 '템플릿' 이미지를 찾는 것이다. 템플릿 이미지를 입력 이미지에서 슬라이딩시켜 전체 이미지에 대해 매칭하면서 두 이미지의 유사도를 계산한다. 이 때 openCV의 cv2.matchTemplate() 함수를 사용한다. cv2.matchTemplate(image, templ, method, result=None, mask=None) image : 입력 이미지 templ : 템플릿 이미지 method : 매칭 방법. 이에 따라 유사도 계산 방법이 달라진다. result : 매칭 결과 행렬. (W - w + 1) * (H - h + 1) 크기의 2차원 array..
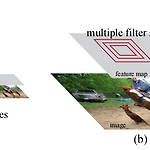
Faster R-CNN: Towards Real-Time Object Detection with Region Proposal Networks https://arxiv.org/abs/1506.01497 Faster R-CNN: Towards Real-Time Object Detection with Region Proposal Networks State-of-the-art object detection networks depend on region proposal algorithms to hypothesize object locations. Advances like SPPnet and Fast R-CNN have reduced the running time of these detection networks,..
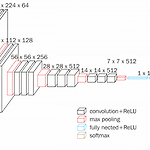
Very Deep Convolutional Networks for Large-Scale Image Recognition * 대규모 이미지 인식을 위한 아주 깊은 컨볼루션 신경망 * Computer Vision 분야에 대한 큰 가능성을 보여줌 * VGG : Visual Geometry Group, 옥스포드대 연구팀 이름 https://arxiv.org/pdf/1409.1556.pdf VGGNet은 CNN의 깊이에 따른 정확도를 연구할 목적으로 만든 이미지 인식 모델이다. Abstract 대규모 이미지 인식에서 convolutional network의 깊이가 정확도에 미치는 영향을 조사 3*3 convolution filter 구조를 사용해 깊은 신경망을 평가 16-19 weight layer의 깊이로 선행..
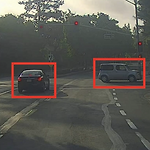
Sliding Windows Detection 이란 Sliding Windows Detection 방법 Sliding Windows Detection 단점 Sliding Windows Detection 구조 (구현) Sliding Windows Detection 객체 위치를 식별하는 object localization의 하나로, sliding window는 이미지에서 8이라는 객체 위치를 찾고 싶을 때, 그 객체를 찾을 때까지 모든 섹션을 확인해 탐지하는 방법이다. Sliding Windows Detection 방법 위와 같이 이미지에서 자동차 객체를 탐지하려고 한다. 1. 차가 있는 이미지에서 차가 아닌 부분을 자르고 차만 남긴다. 2. 이미지 x에 따라 레이블 y는 차 1, 차가 아닌 경우 0으로 설..
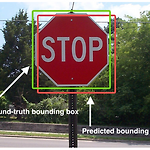
IOU Intersection over Union Ground-truth bounding box : 실제 객체 위치 Predicted bounding box : 내가 찾은 객체 위치 Intersection : 교집합. predicted bounding box와 ground-truth bounding box가 겹치는 부분 (overlap된 부분) Union : 합집합. predicted bounding box와 ground-truth bounding box를 모두 둘러싼 부분 Intersection Over Union (IOU) : Intersection을 Union으로 나눈 비율 즉, IOU는 모델이 얼마나 object detection을 정확히 했는지 정확도를 측정할 수 있는 지표다. HOG + 선형 ..
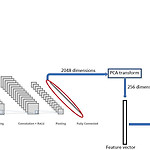
구글이나 네이버 등에서 제공하고 있는 서비스 중, 이미지 검색이 있다. 이미지 파일이나 이미지 URL을 입력하면 유사한 이미지를 검색해주는 서비스다. 이 기술이 Content-based Image Retrieval (CBIR), 즉 컨텐츠(내용) 기반 이미지 검색이고, CNN을 사용한다. 기존의 텍스트 기반 검색이 아닌, 이미지를 구성하고 있는 feature들의 유사도를 기반으로 이미지를 검색하는 것이다. 두 가지 유형이 있다. 1. category-level retrieval 2. instance-level retrieval 예를 들어, 올림픽대교 이미지를 입력했을 때 '다리' 이미지가 어느 것이든 검색되면 category-level, 올림픽대교 이미지만 검색되면 instance-level의 검색이다...